Approved: Fortect
Here are some simple steps that can help solve the problem of correcting english errors.
Mistake 1: Next Sentence Or Comma
The following sentence can be a sentence that connects two independent sentences without proper punctuation or conjunction. A comma is similar to any subordinate clause, but it uses a comma to connect two sentences that don’t have an exact conjunction.
A Few Bug Fixes
Finding grammatical errors isn’t enough until you’re given a suggestion to overcome these problems. Luckily, you’re getting a few bug fixes, giving you the freedom to choose the one that works best with our extended sentence structure checker in English.
2.1″. Other Languages
This paper is dedicated to the task of correcting errors in texts. Most of the research in the area most commonly associated with grammatical error correction (GEC) has been focused on correcting errors made by English learners. A standard approach to solving these problems, which has proved very successful in text- editing contests Et (Dale Kilgarriff, 2011; Dalede plus et al., 2012; Ng et al., 2013; Rozovskaya 2014; et al., 2017), makes full use of the machine learning classifier paradigm, and is also based on a methodology for correcting contextual misspellings (Golding and Roth, 96, 1999; Banko and Brill, 2001). In this particular approach, classifiers are trained for selected types of errors: for example, preposition, article, noun, number (Tetreault et al., 2010; Gamon, 2010; Rozovskaya and Roth, 2010c, b; Dahlmeier and Ng, 2012). .Initially, classifiers were fully trained on English data. When multiple sets of annotated student data became available, models were additionally based on trained annotated student data.
3.1. S2SA-Based Error Correction
A successful error correction model based on the S2SA model has been chosen in this document for the following reasons. (1) The s2sa model is a classic model of modern advances in neural network translation, and its position in the interpretation of neural networks is equivalent to the position of word2vec in the context of text representation. This model undoubtedly introduces an attentional mechanism thatFixes the problem that the decoder can only use this encoder for the final correction of the vector result. This allows the decoder to focus on any important input text to match the next target word. In your component, you can also observe the changes in the new attention weight matrix to find out the sentence text being entered that matches the target phrases. This helps to deepen the understanding of what is related to the model. (2) The idea of all S2SA models is relatively simple, easy to test and understand, their code structure is surprisingly simple, which speeds up model implementation. Even if the base model is not the final model, the problem can be quickly replicated, resulting in unnecessary time savings. (3) The base model is generally easier to use, has relatively few trainable parameters, and can correct data quickly without over-processing. A number of important things are to make searching easier; Unfortunately, most of the bugs found can be more easily identified as bugs.ci found in the data, or the weaknesses of each model. (4) The reference model is useful for data understanding and comprehensive error detection. The process of creating a reference model is very useful for detecting specific deviations and errors in new data. (5) The base model is useful for understanding the problem and understanding which part of the project is the hardest and which is the easiest. According to this idea, it is quite interesting to know what aspect of the business model needs to be improved in order to solve the difficult part in a more profitable way.
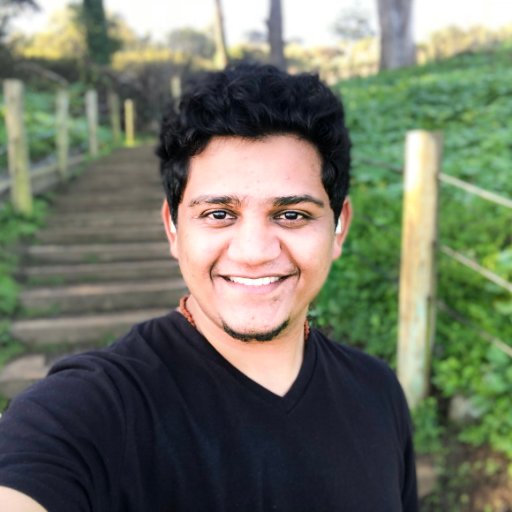