Approved: Fortect
Here are some simple methods that can help you fix your dementia-free audio codec problem.
1. Presentation
Approved: Fortect
Fortect is the world's most popular and effective PC repair tool. It is trusted by millions of people to keep their systems running fast, smooth, and error-free. With its simple user interface and powerful scanning engine, Fortect quickly finds and fixes a broad range of Windows problems - from system instability and security issues to memory management and performance bottlenecks.
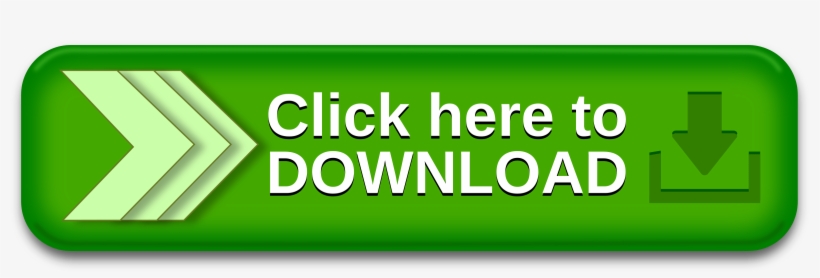
Alzheimer’s disease (AD) is a progressive neurodegenerative disease that affects the lives of more than four million Americans every year. The number of Americans living with AD is expected to more than double by 2050 (Club, 2019).
There is currently no cure for AD (Yadav, 2019), and early detection is essential for effective intervention (Deenken Roeck et al., 2019). Currently ADis being studied using PET imaging and cerebrospinal fluid scans to measure the concentration of amyloid plaques living in the brain, an expensive and therefore costly invasive process (Land & Schaffer, 2020). A much cheaper, non-invasive, and readily available method is AD detection.
Previous studies have shown that language can be used to distinguish healthy patients from AD patients (Pulido et al., 2020). Some researchers have focused on creating new machine learning model architectures for change detection (Chen et al., 2019; Chien de plus al., 2019; Liu et al., 2020) and other used language models (Guo et al., 2019) for AD classification. Indeed, others have focused on extracting acoustic and textual characteristics capturing this information pointing to all ADs. These characteristics include non-verbal characteristics such as the length of the segments and the amount of silence they have (König et al., 2015). Other researchers have used linguistic and melodic characteristics extracted from the English language (Fraser de plus al., 2016; Gosztolya et al. 2019), as well as from the Turkish language (Khodabakhsh et., 2015). Prosodic characteristics were taken from the English language (Ossewaarde et al., 2019; Nagumo et al., 2020; Qiao et al., 2020). Provide acoustic characteristics (Haider et al., 2019). Other researchers need to focus on the specific type of voice generated instead of the model type and function type, and use the concept of human multitasking to increase versatility (Balagopalan et al., 2018). This is a summary of the work that has unfortunately been done over the past few years. A more detailed review of the source literature can be found in the dedicated review de la Fuente Garcia et al. (2020).
Although promising research has been done to date, effective datasets are often imbalanced and differ across analyzes, making it difficult to compare the effectiveness of different methods. Two recent inspection publications (Voleti et al., 2019; de la Fuente Garcia et., 2020) indicate that an important future direction in the detection of cognitive impairment is the provision of balanced and regularThis is a dataset that will enable researchers to understand how to test the effectiveness of various classification and feature extraction techniques. Is this your current attempt at calling ADReSS? The ADReSS task allowed different methods to be applied to the nutrient dataset, eliminating the common pitfalls associated with working with other AD datasets and allowing direct comparisons of these processes.
Previous work may have been done on the ADReSS dataset. Some specialists participated only in the AD classification goal (Edwards et al., 2020; Pompili et., 2020; Yuan et al., 2020), others only participated in the predictive task of the Brief Mental Health Assessment (MMSE) and (Farzana Pardet, 2020) … Alabama., 2020; Rohanyan et al., 2020; Sarawgi et al., 2020; Searle et al., 2020; Syed et al., 2020). Based on Yuan et al. (2020), who applied an 89.6% accuracy of the test, used linguistic features extracted from recordings and coded pauses. Better performance in the MMSE forecasting problem was reported by Koo et al. (2020) who got a root mean square error (RMSE) of 3.747 using a combination of acoustic and text character stick.
In this article, basic sound functions (i vectors and x vectors) and text presentations (word vectors, BERT integrations, LIWC functions, and CLAN functions) have been extracted from data, but used to train classifiers, multiple neural networks. and regression models for detecting AD and then predicting MMSE estimates. I vectors and x vectors, the first that a company should use to validate speakers, have been shown to be effective in detecting AD (López et al., 2019), not to mention other neurodegenerative diseases such as Parkinson’s disease (Botelho et al. , 2020; Moro-Velazquez et al., 2020). Word vectors have also been shown to be useful for detecting AD (Hong et al., 2019). I-vectors, x-vectors, and BERT integrations were used with the ADReSS dataset for AD classification (Pompili al de plus., 2020; Yuan et al., 2020) to predict MMSE estimates (Balagopalan et al., 2020). Pompiliet al. (2020) used the same professional audio equipment features we did and also used BERT integrations, but they did not tie their methods to predicting MMSE problems and their best fusion model was cheaper than yours for Classification problems. Model. The difference between workout and work by Balagopalan also coauthors (2020) and Yuan et al. (2020) is that they just mapped the pretrained BERT creator to the ADReSS data and used what appears to be a model for classification and regression, while my husband and I used the pretrained BERT model as a complete feature extractor and then various trained classifiers regressors and extracted BERT attachments.
CLAN functions have been used in the underlying logs (Luz et al., 2020) and have been used with the BERT integrations in this article to help you determine if scan performance has improved. Finally, the LIWC functions were used to distinguish between AD patients and healthy controls in the scoring (Shibata al et., 2016), but the specific data set was very small (nine AD patients and even nine healthy controls). and our opinion is consistent with In the literature, the use of LIWC for the detection of Alzheimer’s disease is indeed limited. However, LIWC functions were used to analyzefor other aspects of mental health (Tausczik & Pennebaker, 2010) and in general can be useful in the area of AD. For these reasons, we wanted to investigate much more whether LIWC functions could be useful in AD detection and MMSE prediction. While our results do not outperform the incredibly superior performance in MMSE classification and prediction problems, our approaches provide an alternative to previous approaches and provide additional insight into the best AD classification and MMSE prediction methods.
2. Materials And Methods
2.1. Writing ADReSS Data
An ADReSS test record consists of recordings, audio transcripts, and metadata (age including if and MMSE score) for non-AD and non-AD patients. The dataset contains a balanced age, compiled by sex, and the number of patients without AD and AD, 78 patients in each class. Audio recordings are similar to any patient’s recording of the cookie stealing task, where each participant describes what they see in the cookie stealing images. This task has been used for tenYears for the diagnosis and comparison of patients with AD and without AD 1990; (Cooper, Mendez and Ashla-Mendez, 1991; Giles et al., 1996; Bschor et al., 2001; Mackenzie et al. 2007; Choi, 2009; Hernández-Dom Ä ± nguez et al., 2018; Mueller et al. al., al. alabama., 2018), as well as patients with various forms of cognitive impairment and actually screened for aphasia (Goodglass & Kaplan, 1983).
Standard audio tracks have proven their worth
Speed up your computer's performance now with this simple download.
