If you receive a genetic network error message, today’s user guide was created to help you.
Approved: Fortect
Resume
Several studies have focused on the impact of random forest techniques due to their superior performance. Some of these inference methods also have a robust ability to analyze time series as well as determine gene expression data. However, they are only useful for ranking all of their regulations by linking aspects of trust. None of them have been able to discover the specific rules that actually affect the gene most often associated with interest. In this study, we propose this method for removing low-promising candidate rules, essentially combining random forest inference with a number of feature selection methods. As a component for detecting bad rules, our platform uses the results of feature selection procedures to match the confidence values of near-implied regulatory requirements that were actually calculated using inference-based methods. Numerical experiments, which showed that the combined application with feature selection methods improved the use of random forest inference in 98 out of 100 experiments performed on our own artificial problems. However, the improvement is generally small as our method has become collectively popular and removed no more than 19% of possible regulatory requirements. The combo application, in addition to the feature selection process, also helps to increase computational costs. While a larger breakthrough with less computational cost would be ideal, we see no obstacle to our research, as our goal is to extract as much useful information as possible from the limited amount of gene phrase data. PHANTOM5,
Keywords: gene expression, trait selection, aimless forest, genetic network derivation
1. Presentation
The dynamic response of gene expression determines a variety of cellular functions. Our understanding of biological possibilities requires the study of complex patterns of gene regulation, as regulation between your genesand determines how genes are expressed. A safer approach developed for the analysis of inclusive gene regulation is genetic location. In a genetic network task, the general regulation between genes is inferred from gene expression data measured with biological microarray-like technologies to RNA-Seq using slice sequencers. Next age, etc. Derived team models can ideally serve as tools to help biologists create hypotheses and plan their experiments. Therefore, many researchers have been interested in the influence of genetic networks.
A number of methods for the derivation of genetic systems have been proposed (Larrañaga et al., 2006; Meyer et al., 2008; Chou and Voit, 2009; Hecker et., 2009; Matos de Simoes and Emmert-Streib, 2012; Emmert-Streib et al., 2012; Glass et al., 2013). Among these, random forest exposure methods promise excellent performance (Huynh-Thu et al., 2010; Maduranga et al., 2013; Petralia et., 2015; Huynh-Thu and Geurts, 2018; And kimura al., 2019). … Some of these inference models can also compare both time series and static gene expression data (Petralia et al., 2015; Huynh-Thu and Geurts, 2018; Et kimura al., 2019). Data temporarilyThe th series are a series of sets of gene expression levels measured at successive time points after stimulation. Static numbers are sets of levels of gene expression under stationary conditions. Forest-based random inference processes expression data from gene analysis by assigning match values to all candidate protocols. While many genetic inference network methods seek to find the rules that are actually contained in the target network, random forest-based hardware only ranks candidates, assigning a trust value to almost every candidate. When biologists fired to conduct experiments to confirm putative regulatory genes, confidence values calculated by random forest-based methods could have previously been used to determine the order of experiments. However, inference methods based on random forests would be more useful if they had this special ability to recognize the genes that actually dictate the gene of interest.
Combining all the inference methods Based on random forests with a trait selection program, we were able to identify the constraints that are actually contained in the genetic network. Feature selection, a technique studied in computational intelligence, removes variables relevant to the output in a good fit or classification problem (Guyon and Elisseeff, 2003; Cai et al., 2018). However, in preliminary experiments, we have found that a combination method that combines a random forest based capability with one of the existing feature resolution methods often fails to identify the genes that have the weakest effect on the gene of interest. The main focus of existing characterization line methods may explain this failure, as some methods are not designed to identify each of the inputs on which the variables actually affect the output, but to look for inputs through variables that will improve the performance of the output. forecast to maximize this resulting model. Our group recently developed a new feature selection method that aims to find all the functionsRecommended variables that really affect the result, as well as remove as many unimportant input variables as possible (Kimura and Tokuhisa, 2020) / p>
In this manuscript, we propose a method for eliminating less promising candidate positions by combining a random forest inference method with the innovative feature selection method we developed in Kimura and Tokuhisa (2020), as well as two adapted versions. Characterizing the methods used in this study typically involves not only removing a few irrelevant contributing variables, but also assigning confidence to the input variables to show the likelihood that a person actually influences their use. In our combined method, we can use the confidence values computed by all feature selection methods to match the probability values assigned to all n candidate rules using the random forest method.
The other in this manuscript is structured as follows. In section 2, we present the logicalinference based on a random forest used in this study. In section 3, we describe the methods for choosing the appearance and explain the possibility of combining them with the secret of the output. We confirm the effectiveness of the combined method indicated by numerical experiments using spurious and biological gene expression data in sections 4 and 5. Finally, this will be concluded in section 6 with the future work of our company. / P>
2. Inference Method Based On Random Forest
As previously mentioned, this study combines the influence of the random forest method with a number of approaches to feature selection. While this can be done with any random forest-based method, in this study we use an inference method (Kimura et al., 2019) capable of analyzing both time series and static gene expression data. The section of this proposal describes the withdrawal method.
2.1. Model For Describing Genetic Networks
The inference method used in a particular study describes a genetic network that uses each set of differential equations, often having th form
where X −n (X 1 , = â ‹¯â € ‰, X n−1 , X n + 1 , â ‹¯â € ‰, X N ), X m (m = 1, 2, â‹ ¯â € ‰, N ) is the expression level of the mth gene, N is the number of genes contained in the target network, Î n (> 0) is a constant parameter and, therefore, F n is a function random shape.
Using this model, we get a legacy network, getting the function F n in addition to the parameter β n (n = 1, only â ‹¯ …, N ), which cause sequential changes over time with respect to the observed levels of gene expression. The corresponding section is one of the ways to secure them.
2.2. Derived From F N And β N
The Effects method (Kimura et al., 2019) divides the inference condition of a genetic network consisting of N genes into N subtasks, each of which corresponds to each gene. Solving your n-th subproblem, the method obtains a conservative approximation of the function F n and the best reasonable value for the parameter β n . The remainder of this section describes the new nth subproblem.
2.2.1. Identifying the problem
Method effects used in this studyIn the study, have a significant approximation to the function F n and an increased value of the parameter β n thr.
Approved: Fortect
Fortect is the world's most popular and effective PC repair tool. It is trusted by millions of people to keep their systems running fast, smooth, and error-free. With its simple user interface and powerful scanning engine, Fortect quickly finds and fixes a broad range of Windows problems - from system instability and security issues to memory management and performance bottlenecks.
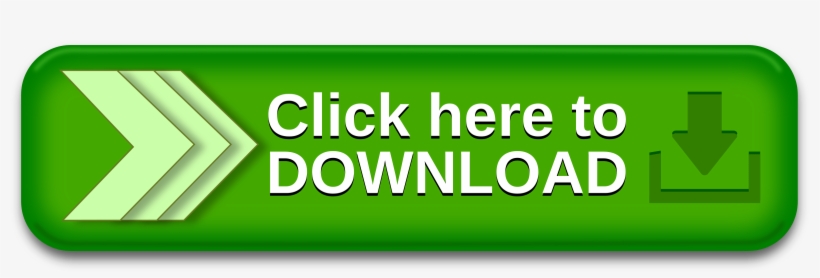
Speed up your computer's performance now with this simple download.
