Approved: Fortect
Here are some simple steps that should help you fix the core component’s core problem.
Usually in the field of multivariate statistics, the main component of the analysis (core PCA) [1] is a Critical Component Analysis (PCA) extension that uses methods rather than kernel methods. When using the kernel, initially simple PCA operations are performed in any type of Hilbert replay space in the kernel.
Context: Linear PCA
Remember that traditional PCA works with center-zero data; your
-
,
where
multidimensional observations.Diagonalizing the covariance matrix alt = “C = frac,
in other words, it allows you to automatically decompose the covariance matrix:
-
. [2]
Introducing The Core To PCA
Approved: Fortect
Fortect is the world's most popular and effective PC repair tool. It is trusted by millions of people to keep their systems running fast, smooth, and error-free. With its simple user interface and powerful scanning engine, Fortect quickly finds and fixes a broad range of Windows problems - from system instability and security issues to memory management and performance bottlenecks.
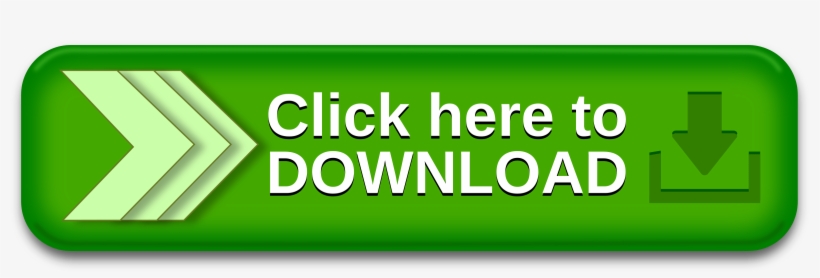
To understand the utility described by all PCA kernels, especially for clustering, note that with respect to N points, usually non-linear in Dimensions. This means given N-details , and if we map them to an open space with N dimensions
-
where
,
Easy to buildfind a hyperplane dividing points into arbitrary clusters. Of course,
creates linearly independent vectors, there is no covariance and self-expansion is explicit, since we support linear PCA. Instead, the PCA core has a great function is definitely “selected” which is never explicitly evaluated, which allows this possibility
as never before have we really needed to define data in this area. Since we can usually try to avoid working in a certain space we are going to get space ‘,’ functionality with which we can create a specific core N-by-N
which It is a manifestation of the internal product space (see the Gram matrix) in an otherwise stubborn repository of features. The dual form that occurs during a certain kernel generation allows us to mathematically formulate a version of PCA in which we never add my eigenvectors and covariance matrix eigenvalues around
-space (see kernel tip). The N elements of each K column usually represent the dot product of a human transformed data point to uniquely account for all transformed points (N points). Some popular popcorn kernels are shown in the following example.Since I never work directly in the area of performance, the formulation of the core PCA is prohibited, since it calculates not the main parts, but the projections of human data onto these components. To evaluate your projection from a point in aspect space
in the k-th mainM component (where exponent g means component k, not exponent k) Please note that most denotes a dot product that simply consists of core elements
. It looks like whatever is open can be computed and normalized
Speed up your computer's performance now with this simple download.
Kernel PCA uses the core function of the design dataset in the higher-dimensional function space, where it can be divided linearly. This is similar to the idea of support vector machines. There are various Kernel systems such as linear, polynomial and Gaussian.
Basic ingredients are new things that are built as linear combinations with mixtures of the original variables. Geometrically, the principal components represent the data directions that explain the maximum dose of variance, that is, all the rows that capture most of the information in your current data.
Machine Learning (ML) Core Principal Component Analysis (KPCA) is a special method of nonlinear dimensionality reduction. It is an offshoot of Principal Component Analysis (PCA), which is unfortunately a linear dimensionality reduction tactic using kernel methods.
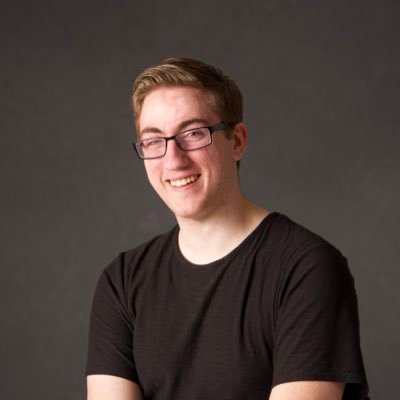